Why Manufacturers Need a Phased Approach to Digital Transformation
Those that succeed with this difficult work break it into three stages, each with its own guiding metrics.
News
- AI Research Forum and Summit Focused on Agentic AI Announced
- Cisco's New Quantum Entanglement Chip Aims to Accelerate Distributed Computing
- 80% of Middle East Cyberattacks Result in Data Breaches, Warns New Study
- Global leaders convene in Abu Dhabi for inaugural Governance of Emerging Technologies Summit
- Gulf Nations Fast-Track AI Ambitions, UAE Leads Regional Readiness, says BCG Report
- AI Professionals form a Redefined Workforce. But Systemic Roadblocks Persist, Survey Finds
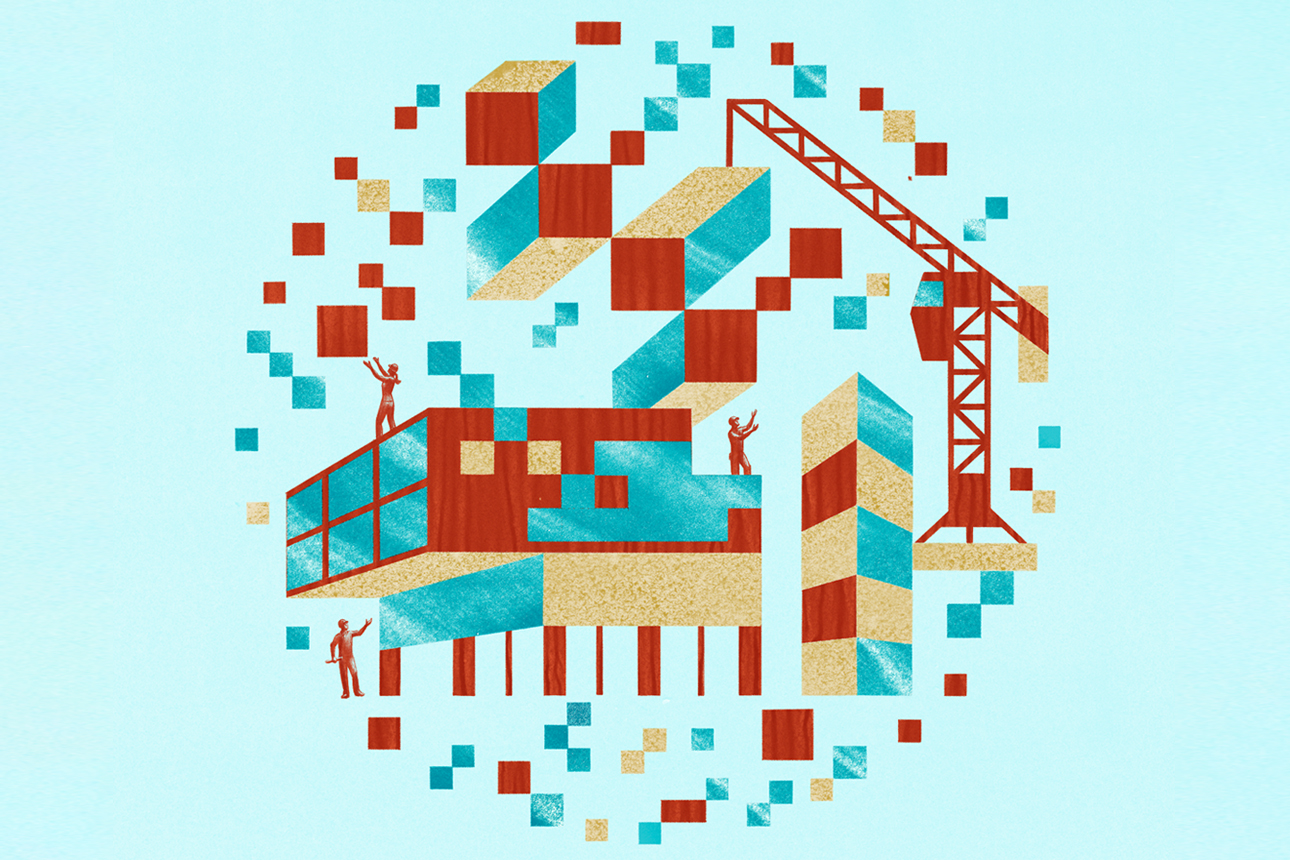
Alex Nabaum/theispot.com
As complex as digital transformation can be for manufacturers, leaders in that sector tend to view it as a single process, the success of which can be demonstrated via a single metric: return on investment.
Such a simplistic view belies what’s actually involved: investing in and mastering new operational technologies (OTs), reskilling workers, keeping capabilities in sync with external supply-chain partner infrastructures, and enabling new digital ecosystems for partners and customers. Digital transformation for manufacturing differs substantially from transforming IT services or implementing e-commerce, because it requires combining the staged integration of physical assets with digital technologies. For these and other reasons, many manufacturers struggle to adopt transformative tech and end up misaligning and wasting their investments or misdirecting their scarce specialized resources. As a consequence, their digital investments generally fail to enable the business transformation they seek.
Our studies find that manufacturers’ digital transformation journeys involve three distinct stages, each with its own set of appropriate metrics. The companies that correctly identify where they are in this work and then apply the right metrics are more likely to achieve the kinds of innovation they seek. Given the huge investments manufacturers are making in digital innovation and transformation, the stakes are extremely high. A failure can waste enormous capital investments, put competitiveness at risk, and cost many people their jobs. That might be why some leaders fall back on traditional ROI measures. However, those metrics provide a false sense of security — and they do nothing to help managers reach the advanced stages of digital transformation that promise benefits far more strategically valuable than mere cost savings and productivity gains.
Applying the wrong metrics — that is, those more appropriate for a different stage — can be a costly mistake. One glaring example is Predix, General Electric’s industrial internet-of-things edge-to-cloud platform for digital applications, which was designed to collect both OT and IT data and then transfer it to the cloud. However, by working with the wrong metrics — namely, revenue growth and the number of developers the platform attracted — GE not only overinvested in Predix but also failed to develop the right metrics on initial market needs and response. For example, GE measured revenue too soon and, worse, measured existing revenue rather than new revenue. The company predicted that Predix would deliver revenue of $6 billion for the 2015-16 period, but the actual reported figure was closer to $1 billion, according to contemporaneous reports. Revenue is not a good measure for new, innovative projects, which can take time to develop. Promising too much, too soon — as GE did — can foster unrealistic expectations, and when those expectations are inevitably unmet, the result is often a project’s drawdown or even cancellation.
Similarly, GE misapplied a metric around developers. The Predix team managed to sign up some 22,000 developers, but GE neglected to consider an equally important metric: developer engagement. Predix’s level of developer engagement was extremely low, with developers reportedly creating a mere 150 applications. As of 2016, the total cost to GE from the Predix market mishap was estimated at $5 billion, a sum The Wall Street Journal described as “massive … even by GE standards.” More recently, GE has refocused its overall business on aerospace and energy; the digital group, of which Predix was a leading product, has been quietly tucked away.
In contrast, tracking the right metrics allows managers to check progress toward their current goal in rigorous and objective ways so that they can make course corrections and keep moving in the right direction.
Understanding the Three Stages of Digital Transformation
In our studies of manufacturers undergoing transformation, we’ve observed that successful initiatives follow a typical three-stage arc. In Stage 1, the focus is on point solutions that leverage technology to cut costs and boost productivity. In Stage 2, leaders turn toward developing organizationwide digital infrastructure and capabilities, which are the necessary foundation for Stage 3, where the company begins to deploy technology in service of its strategic ambitions.
While the successive stages typically build on one another, a manufacturer’s movement is not always linear or sequential: Manufacturers might have discrete Stage 1 projects underway while also pursuing Stage 2 infrastructure work and exploring Stage 3 strategic work simultaneously. That said, most legacy manufacturers will require a major overhaul of their IT infrastructure in order to scale Stage 1 projects or execute ambitious Stage 3 work. (See “Digital Transformation’s Three Stages.”)
Digital Transformation’s Three Stages
Each stage of digital transformation requires attention to a different set of stakeholders and should be measured against a different set of key performance indicators.
Next, we’ll look at each stage and its associated metrics in depth.
Stage 1: Gain efficiency. This stage of digital transformation involves projects internal to the manufacturing company that are meant to achieve localized productivity gains realized as point solutions. Promising technologies are generally piloted at this stage for potential benefits at either individual factories or value chain points. For example, a manufacturer of electronic components might take a process previously done manually and automate it with industrial robots.
Here, KPIs tend to be based on well-understood measures of ROI, such as lowered costs, faster payback periods, higher quality and yield, and increased revenue. Metrics evaluating progress at this stage might also measure product improvements, productivity gains, and employee engagement. If a project makes a manufacturing process faster and/or cheaper, then the project can be said to have met its Stage 1 goals.
An initiative by consumer goods giant Unilever to improve the process of manufacturing powdered laundry detergent provides an example of such a project. Historically, the process has begun by spraying a slurry of ingredients into a special-purpose tower. This slurry is then blasted with hot air, which dries the slurry to a powder. It’s a process traditionally controlled by factory operators, who ensure that the powder is neither underdried nor overdried. This task is often deemed a bit of an art, requiring an expert operator to constantly monitor the drying process and make frequent adjustments.
Unilever saw this process as ripe for automation, and the COVID-19 pandemic accelerated the initiative as the factory control room — where the towers were monitored 24-7 — did not allow for adequate social distancing. At two of its facilities in South America, the company developed automated towers equipped with machine learning algorithms that simulate different manufacturing scenarios and predict outcomes. In Africa, Unilever used this capability to remotely start up a powder tower after the operators had been sent home due to the pandemic. These insights from the application of machine learning, based on real-time data, were then delivered to technicians via a digital dashboard that could be accessed not only onsite but remotely, from a home office.
The metrics Unilever used to keep the project on target included:
- ROI via lower operating costs and better energy efficiency.
- A higher product yield and more consistent product quality, based on improved moisture control and measurable powder properties.
- A shorter payback period to recover investment costs.
- Improvements in energy, materials, labor, and asset productivity.
Following the success of its initial South American project, Unilever is now rolling out the intelligent powder tower innovations to its production sites worldwide. And it has taken its intention to embark on a Stage 2 initiative by shifting to a cloud-only IT infrastructure.
Stage 2: Build new digital capabilities. The overarching goal driving Stage 2 digital transformation is building functional capability to enable seamless data flows within and outside of the organization. At this stage, companies put in place the digital infrastructure required for advanced data analytics, end-to-end supply chain integration, and digital connectivity with partners and customers.
Participants in these projects will be mostly from within the company, but external technology providers and business partners are often involved as well. Stage 2 projects often involve the integration of OT with IT, and this can require organizations to gain advanced skill sets in both areas. They also frequently require companies to significantly improve data governance and break down functional silos that impede data access across the company.
Typical Stage 2 metrics for evaluating project success include:
- The time needed to create a new capability, monitored routinely against interim milestones. Resources such as labor are also monitored.
- The efficiency of a new or an enhanced system capability for a defined operational function, and its level of adoption, as measured by internal and external stakeholder engagement. This includes usage numbers, the ratio of positive to negative feedback, and other communications data.
- The time needed to implement the new capability across the product portfolio and supply network.
- The number of related applications and innovations, or augmented solutions, that are built atop the new infrastructure.
Schneider Electric, a 180-year-old manufacturer of electrical equipment that serves customers worldwide, is pursuing a companywide effort to create a unified set of software, a common user experience, a software layer enabling universal access to heterogeneous data sources, and AI capabilities. As might be expected from a Stage 2 initiative, Schneider is seeking benefits that go far beyond mere cost cutting. “Not everything comes back to ROI,” the company’s vice president of global supply chain performance told us. “A value-creation mindset encapsulates both tangible and intangible needs from a broader set of stakeholders.”
In contrast to Stage 1 projects — which are often planned with a clear start, middle, and end — many of Schneider’s Stage 2 projects are executed in short, intense sprints. This tactic aims to avoid the cost escalations, benefit delays, and participant dropouts that often plague long-term IT projects and can lead to their failure and even abandonment.
This also means that at the start of a Stage 2 project, Schneider doesn’t focus on the project size, cost, or ROI. Instead, the company monitors progress continually, typically checking in after each sprint is completed. “You start with a vision, then work backward in buckets,” the supply chain executive said. For instance, one such project is Schneider’s new supply-chain control tower. This function integrates nearly 1,000 inputs — some from in-factory IoT sensors, others from Tier 1 suppliers or customers — across multiple manufacturing plants. The tower gives Schneider complete, continuous visibility into all orders while they’re in transit. It also provides an alert-management system that uses predictive software to detect system disruptions and delays and then correct them with self-healing capabilities.
To measure and monitor the control tower project, Schneider tracked several Stage 2 KPIs. These include:
- Control tower visibility: Visibility into both inbound and outbound shipments was continuously measured to monitor the percentage of schedule variations in inbound materials and outbound product orders currently in transit across multiple plants and distribution centers.
- Customer engagement and service-output capability: The control tower’s ability to enhance customer service levels is measured based on the number of system alerts generated in anticipation of supply issues, and on a reduction in the number of orders unfulfilled, postponed, or canceled. This also includes the development of new capabilities, such as causation analysis; identification of the percentage of components at risk; a reassessment of safety stock levels (inventory days); and measures of both customer-service levels (based on schedule adherence for “on-time in-full” metrics) and customer satisfaction (based on surveys).
- Control tower coverage across products and the supply network: This refers to the rate at which the alert-management system detects system input disruptions and delays across the entire product portfolio and the manufacturing and supply chain footprint.
- Future development of autonomous planning and self-healing capabilities: This involves leveraging the new control tower capability to develop the next generation of solutions, such as autonomous planning and self-healing (automated corrective) processes.
Taken together, these KPIs help Schneider anticipate issues before they become problems and ensure that customers receive their orders accurately and on time. Therefore, Schneider has a key set of Stage 2 metrics around customer engagement and service-output capability. The value being created is not mere ROI but rather Schneider’s ability to attract new customers, serve them reliably, and earn their loyalty.
Stage 3: Grow via digital innovation. Modernizing IT infrastructure and developing new digital capabilities enables growth through new business models — a critical need for manufacturers. This typically means robust digital engagement with partners and customers, such as by establishing and operating new digital ecosystems that add value for customers via partners’ complementary offerings, or operating within digital ecosystems orchestrated by others.
Participants in this stage include both internal and external players, such as technology or service providers and customers. While Stage 1 and Stage 2 KPIs are primarily internal, Stage 3 metrics look both inward and outward for measures of success.
For a platform/ecosystem project, these Stage 3 KPIs can include:
- The number or percentage of ecosystem partners engaged.
- The number or percentage of new customers attracted.
- The adoption rate — that is, how quickly existing customers switch to the new platform.
- Transaction volumes occurring on the new platform.
- New types of transactions occurring on the platform.
- The number of new sources and types of interactions enabled by the platform.
One form of Stage 3 innovation is being executed by apparel makers to transform the customer experience. Among these manufacturers is Ralph Lauren, a company with $6.2 billion in revenue that has offered luxury lifestyle products, including clothing, bedding, bath products, and floor coverings, for more than a half-century. Today, one of Ralph Lauren’s top strategic objectives involves the adoption of digital identity technology. This approach assigns a unique serial number to each clothing item at its time of manufacture. Consumers can then use their smartphones to scan the digital identifier on the item’s label to learn where it was made, read consumer reviews, and find out whether a store stocks the item in their size. They can also confirm its authenticity — important in a market segment plagued by counterfeit goods. Later, consumers can use the same digital identifier to submit their own reviews, collect loyalty points, and receive focused brand content — increasing engagement and giving Ralph Lauren a direct channel to its customers.
In addition to measuring its ability to track products throughout their entire life cycle for supply chain and marketing insights, Ralph Lauren is using both internal and external metrics to guide its Stage 3 work. These include:
- Brand integrity and trust: The number, brands, and types of products that consumers can authenticate on their own.
- Consumer engagement: The rate at which consumers scan for product information, brand, and style content.
- Partner ecosystem: The number of new partners and new types of partners attracted.
- Factory activation: The number of garments equipped and activated with digital serial-number labels.
Ralph Lauren has applied the digital identifiers to tens of millions of products, starting with its well-known Polo brand. Behind the scenes at the company, a blockchain-based digital distributed ledger system enables close monitoring of both manufacturing inputs and outputs. For example, the company can now track the number of daily digital identifier activations by brand. The blockchain also captures information on the top locations worldwide that are producing counterfeits of its products, helping the company focus its product authentication efforts. Sales of individual products are easier to track, too, providing Ralph Lauren with actionable supply chain and marketing insights.
Adopting a Staged Transformation Approach
For manufacturers seeking to match their metrics with the appropriate stage of digital innovation and transformation, we offer the following recommendations.
1. Determine which stage an individual innovation project is in, and then involve the appropriate stakeholders and champions, and adopt the right metrics.
Issue: Like most large manufacturers, you’re probably engaged in multiple projects.
Implementation mechanisms: Limit your investigation to a single business process, business unit, or location. Then determine the project’s focus and goals. Is it a Stage 1 internal point solution? If so, then you can apply ROI and other cost-related measures. A Stage 2 technology-enabled functional capability? Then your metrics should be charting the capability’s inputs and outputs. Or is it a Stage 3 external-facing market transformation? In this case, your metrics should involve measuring the participation of others — including customers and business partners — outside the organization.
2. Use your data as you make progress.
Issue: Data sets are constantly evolving in manufacturing settings. The right data can help you gain buy-in from important board members, update your metrics, and even select new and more relevant metrics along the way.
Implementation mechanisms: One best practice is to avoid the kinds of multiyear projects, complete with long-term metrics, that often end in either disappointment or a failure to be completed at all. Instead, set goals for the short term — say, three to six months — and measure them with equally short-term metrics. A positive review will give you the green light for the next stage. And if the review highlights one or more problems, you still have time to make an early course correction, avoiding a costly and possibly job-risking failure.
3. Don’t join the liar’s club.
Issue: Even when ROI is not the right metric for a digital innovation project — and often it’s not — project leaders are tempted to use it.
Implementation mechanisms: Don’t propose myopic ROI metrics merely to gain early support from your financial teams. While it’s true that ROI is a basic concept your CEO, CFO, and other board members already understand, pretending to care about ROI can be a wily way to gain time. But remember: ROI is a Stage 1 metric, meaning your gains might be incremental. Assuming that your project sits in Stage 2 or 3, it’s far better to agree with your key stakeholders on alternative metrics for measuring success instead. Depending on which stage your project is in, these metrics could include the creation of new capabilities and options, delivery speed, the risk of not implementing the system, and how a project aligns with the overall corporate strategy. This might require you to educate stakeholders about why metrics other than ROI are more appropriate for your project. But your upfront investment of time will be paid back quickly, and with interest.
We’ve seen how the inherent complexity of a manufacturing business can complicate, slow, or derail digital transformation efforts — and we’ve seen the considerable benefits that digital technologies can bring to manufacturers that are able to chart a well-planned path to implementation. Manufacturers that follow the staged approach that we suggest here — which emerged from our close study of exemplary companies — should, like those businesses, be better able to thoughtfully build new digital capabilities with the right leadership skills to keep projects on track through the disciplined use of relevant metrics at each stage.
Keen to know how emerging technologies will impact your industry? MIT SMR Middle East will be hosting the second edition of NextTech Summit.