Responsible AI: Balancing Innovation and Trust
Experts say fostering trust between users and society is the foundation for responsible AI innovation.
Topics
NEXTTECH
News
- Dubai Future Foundation and WEF Release Top 10 Emerging Technologies of 2025
- Deloitte and AWS Forge $1 Billion Alliance to Accelerate Digital Transformation in the Middle East
- Over 40% of Agentic AI Projects Expected to Fail by 2027, Report Finds
- Guardian AI Agents Poised to Take 15% of Agentic AI Market by 2030, Gartner Says
- Deloitte Rolls Out Global Agentic Network to Accelerate AI Adoption in the Middle East
- AI Research Forum and Summit Focused on Agentic AI Announced
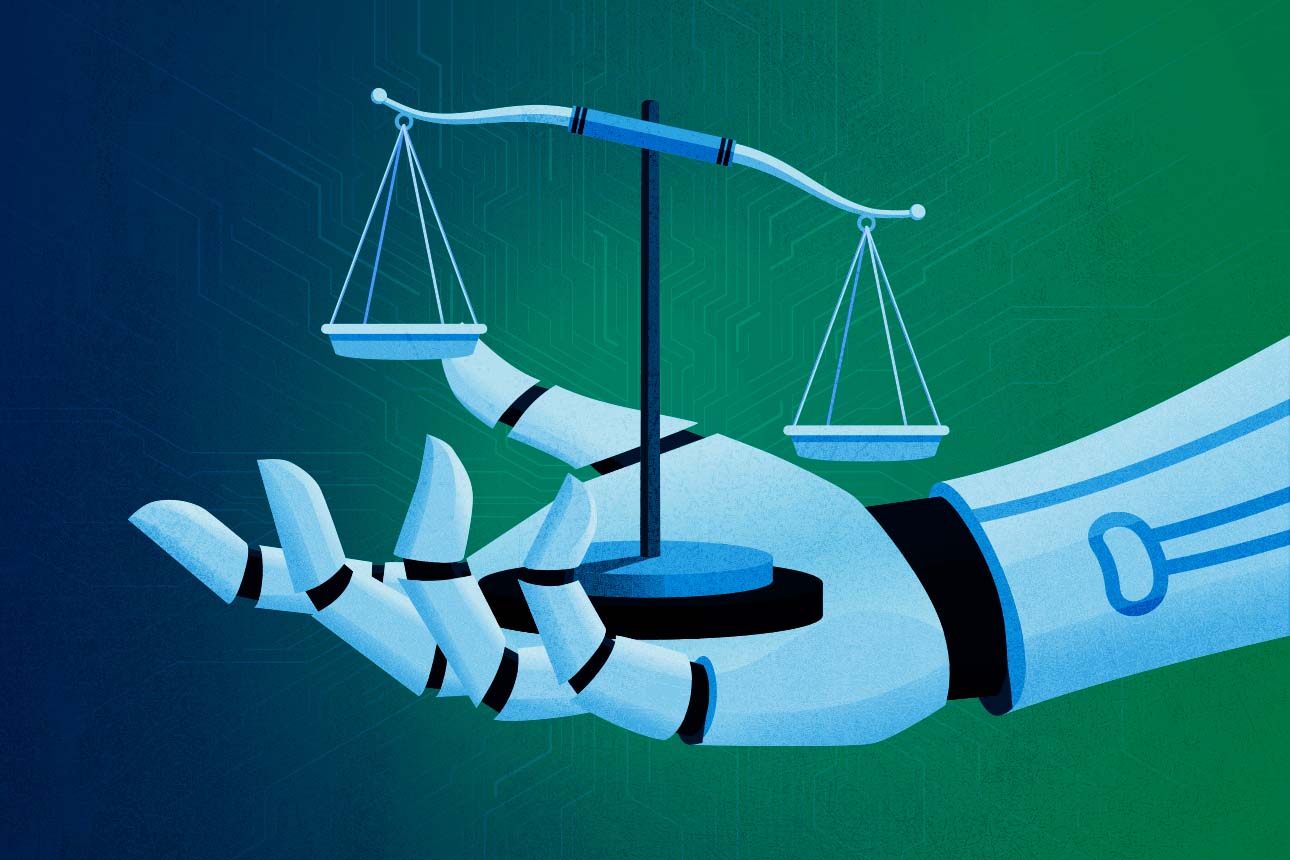
[Image source: Krishna Prasad/MITSMR Middle East]
Dr. Joy Buolamwini, in her book Unmasking AI: My Mission to Protect What Is Human in a World of Machines, released last year, addresses the inherent biases and ethical implications of AI systems. The computer scientist and the founder of the Algorithmic Justice League, who laid the groundwork much before AI became mainstream, in a 2016 TED talk, discussed how face recognition software systems did not recognize her face until she used a white mask, thereby revealing deep-set race and gender biases in these AI systems.
This was only part of the much larger problem, and the discussion was confined to a potentially smaller group of researchers, academicians, and experts at specialized forums, academic conferences, and industry gatherings.
It is only after post-pandemic and the likes of ChatGPT and Gemini (formerly Bard) taking the spotlight all through 2023 that the conversations around transparency, accountability, privacy, inclusivity, and human-centered design principles—all in all, the need to develop responsible AI (RAI)—gained momentum.
Today, there is no denying that AI is the “new normal,” and more and more industries will see the adoption of the technology in the near future.
To understand how companies in the Middle East region ensure accountability, build trust and confidence when putting models into production, and address the barriers to achieving responsible AI, MIT SMR Middle East reached out to top leaders in the region. In this article, Saad Toma, General Manager of IBM Middle East and Africa; Celal Kavuklu, Head of Pre-sales at SAS, META; Sandeep Dutta, Chief Practice Officer at Fractal.ai, APAC; and AI/ML Senior Manager at Acronis, Chan Wah Ng, share their views.
1. Ensuring accountability throughout the development and deployment lifecycle of AI systems: While it’s clear that the transformative potential of AI will be the driver of immense change, it is not yet clear whether the benefits of AI will be broadly shared or controlled and doled out by just a few big stakeholders. To guarantee that all can harness the transformative changes of AI is to ensure that the “future of AI is open,” says IBM’s Saad Toma. Global governments need to focus on risk-based regulation, prioritize liability over licensing, support open-source AI innovation, and ensure proactive corporate accountability to make sure that AI is explainable, transparent, and fair.
From data collection to analytics to decision-making, individuals and organizations must recognize their role in systems across the lifecycle. “Accountability is a shared responsibility of all people and entities that interface with an AI system,” adds Kavuklu of SAS, an AI and data analytics firm. The key is identifying and remedying issues, learning from feedback, and ensuring proactive measures to mitigate adverse impacts.
Sandeep Dutta of Fractal.ai, advocates for a human-centric approach. This includes incorporating behavioral nudges to prioritize fairness and ethics, alongside human oversight and modular AI design for better risk mitigation.
Integrating AI-specific safeguards by implementing an RAI toolkit that addresses privacy and safety, fairness and equity, accountability, and transparency, throughout the lifecycle is essential.
2. Greatest barrier to achieving responsible AI: IBM’s annual Global AI Adoption Index recently found that while 42% of enterprise-scale companies have deployed AI in their business, 40% more are exploring or experimenting with AI but have not yet deployed their models.
When companies and the top leadership struggle to adopt GenAI, their first concern isn’t the tech talent pool or other organizational obstacles; it is more basic: data. Without reliable data, even the best AI will deliver faulty, biased, or dangerous results. The top barriers to this are data lineage and provenance, a lack of proprietary data to customize, and security concerns.
Another hurdle is the lack of prioritization within organizations. While many organizations acknowledge the importance of responsible AI, it often doesn’t occupy top priority. “Do you have a well-defined RAI framework implemented with a senior person clearly responsible for it? If I ask this question to 10 organizations, not more than three will answer yes or be able to demonstrate it today,” says Dutta. “So, the litmus is not merely recognizing the importance of RAI but actually making it a mandatory requirement.”
The complexity and novelty of AI technology, coupled with the lack of well-defined regulations and ethical frameworks governing its development and deployment, is also a major factor. Additionally, Kavuklu highlights the inherent biases in AI algorithms and the challenges of ensuring human oversight further contribute to the barrier.
Acronis’ Chan Wah Ng echoes similar sentiments on the lack of an established framework. “We have to get through by accumulating test cases as we develop and run the GenAI applications. In terms of ethical AI, we rely on the providers of such foundation LLM models since we are not the ones who trained the model. As a precautionary measure, we set up guardrails and filters to check on the input/output to/from the LLM, to ensure the data generated are safe.”
3. Building trust and confidence when putting models into production: Trust in AI hinges on a deeper understanding of how AI models are trained, with what data, how they arrive at their recommendations, and whether they are routinely screened for harmful and inappropriate bias. “We don’t regulate the wheel, but rather its use on cars, trains, and airplanes. The same approach is the right one for AI,” says Toma.
And building trust is a challenging task. The process involves several key aspects, such as transparency in methodology, honesty about uncertainties, clear communication, and demonstrating competence in model development and application. Users need to understand how the model works, what data it uses, and the level of certainty associated with its predictions. Kavuklu says “acceptance is crucial” for successfully implementing and utilizing AI and predictive models in various domains, including energy management, finance, healthcare, and more.
“Until very recently (i.e. pre GenAI models), our AI models are mostly classifiers and detectors. So, we can build up trust by having extensive test sets, and setting up requirements that mandate accuracy at a specific false-positive rate. With these test results, we can be fairly confident in the models,” says Ng. Such kinds of AI models are also pretty straightforward to explain, as there are established techniques such as Local Interpretable Model-agnostic Explanations (LIME ) and SHapley Additive exPlanations (SHAP) to help us understand why a model arrives at a particular decision given the input. With GenAI, it is a different story, Ng adds. “By its nature, the output of GenAI models will differ from one run to another, even with identical output.”
The long-term advantages of AI for both business and society are undeniable. In fact, AI is our best hope for solving some of the most pressing challenges ahead of us in areas like healthcare, agriculture, education, and sustainability, as well as solving the challenge of increasing productivity needed to fuel the world economy, adds Dutta.
As the world grapples with the multifaceted challenges of responsible AI development, proactive engagement and adherence to ethical frameworks remain indispensable in ensuring that AI serves humanity ethically and beneficially.
More importantly, fostering trust between users and society is the foundation for responsible AI innovation. As Buolamwini writes in her book, “AI should be for the people and by the people, not just the privileged few.”
At the NextTech Summit, the region’s foremost summit focusing on emerging technologies, global experts, MIT professors, industry leaders, policymakers, and futurists will discuss AI Black Box, Quantum Computing, Enterprise AI among many other technologies and their immense potential. The summit, with Astra Tech as Gold Sponsor, will be held on May 29, 2024.