Open Data's Potential for Innovation Hinges Not Just on Accessibility But on Usability
Experts say making data accessible is an essential first step, but ensuring it is usable involves several additional considerations.
Topics
MIT SMR CONNECTIONS
News
- Deloitte and AWS Forge $1 Billion Alliance to Accelerate Digital Transformation in the Middle East
- Over 40% of Agentic AI Projects Expected to Fail by 2027, Report Finds
- Guardian AI Agents Poised to Take 15% of Agentic AI Market by 2030, Gartner Says
- Deloitte Rolls Out Global Agentic Network to Accelerate AI Adoption in the Middle East
- AI Research Forum and Summit Focused on Agentic AI Announced
- Cisco's New Quantum Entanglement Chip Aims to Accelerate Distributed Computing
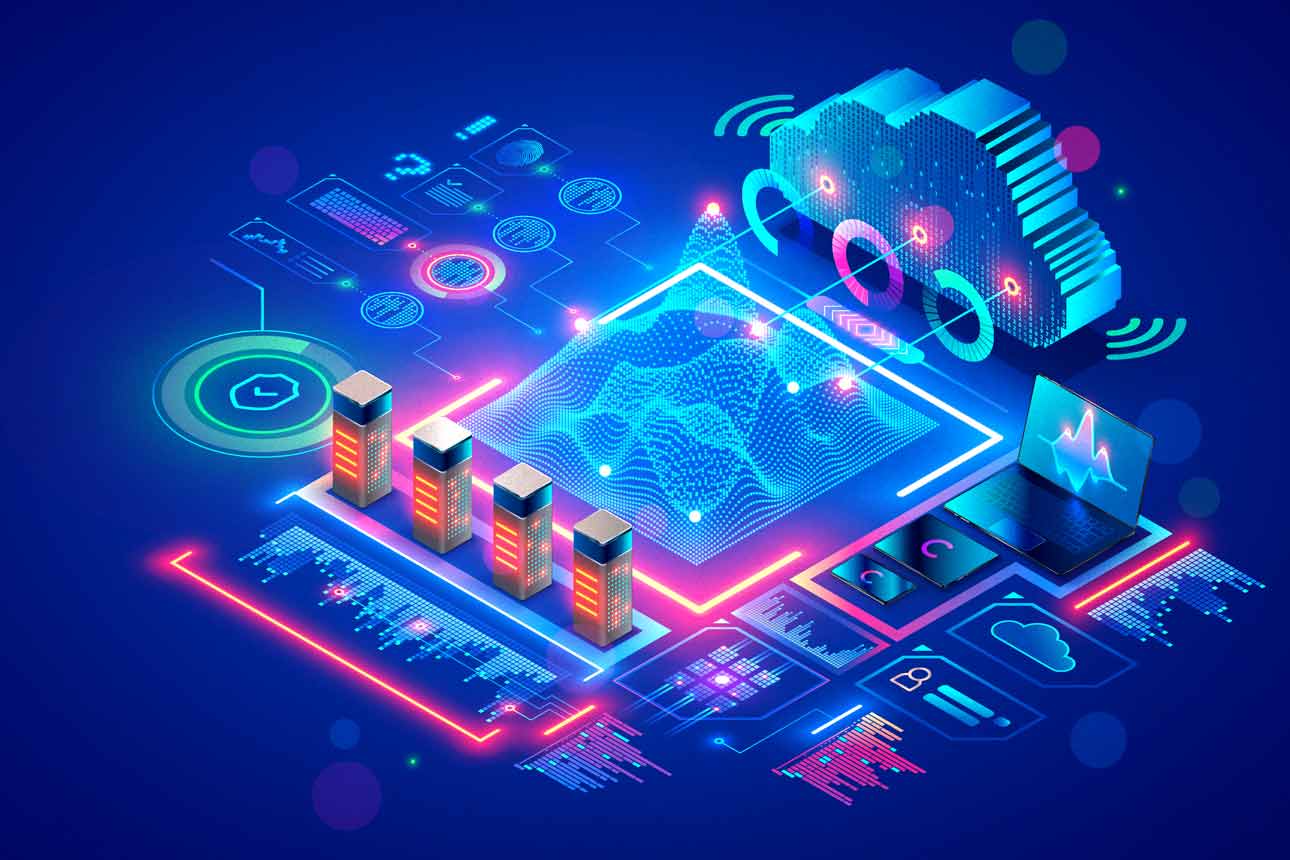
[Source photo: Krishna Prasad/Fast Company Middle East]
The data landscape has become immense and complex, and the explosion of data has made it increasingly challenging to extract value from it.
Open data, accessible public data that people and businesses can use to launch new ventures, analyze patterns and trends, make data-driven decisions, and solve complex problems, is essential to the burgeoning data landscape. Businesses across industries use relevant open data to improve their products and services.
However, as time passes, the quantity of open data is becoming vast, and it keeps gaining momentum, with more and more people pouring their data into the online space. The internet is flooded with an ever-increasing amount of disparate information.
So, although open data has been hailed as a catalyst for innovation, there are attendant challenges—how can we integrate diverse forms of open data? How are data points interconnected? How can open data be cross-checked across different sources to provide valuable insights? Finally, does accessibility guarantee usability?
“Open data’s potential for innovation hinges not just on accessibility but also on usability, influenced by many different patterns such as data quality, format, metadata, standardization, licensing, discoverability, user skills, and last but not least community support,” says Fred Crehan, Area Vice President Growth Markets at Confluent.
High-quality, machine-readable data with comprehensive documentation and clear licensing facilitates use. Standardization promotes interoperability, while effective discoverability connects users with the data they need.
However, Crehan adds that the technical skills required to analyze complex datasets and the presence of a supportive community can significantly impact the ability to fully leverage open data. Ensuring usability alongside accessibility is crucial for maximizing open data’s societal benefits.
Open data has applications far outstripping IT and cybersecurity. It could be translated from unstructured open data into structured serviceable intelligence, with data analysis solutions.
Some of the most data-driven organizations have invested in building modern Kubernetes-based data services platforms. “Their data scientists and AI researchers use it to perform data preparation, cleansing and exploration at scale. They recognize that this is an essential phase before using any data, be it open or private,” says Fred Lherault, Field CTO, EMEA Emerging at Pure Storage.
Several Additional Considerations
While making data accessible is an essential first step, ensuring it is usable involves several additional considerations. According to Jad Khalife, Director of Sales Engineering, Middle East & Turkey, Dataiku, usable data must meet the quality requirements of the business, be documented for users to understand the structure, content, and context of the data, and ensure we are ethically using the data, data governance, and ease of data access.
“Accessibility encompasses not just the availability of data but also the ease with which users can find, retrieve, and access it. Users need to be provided with easy-to-use visual interfaces to browse the data,” says Khalife.
While key elements of an open data approach include transparency, collaboration, and accessibility, Crehan says it is essential to ensure that applications that rely on up-to-the-minute data, such as those in public transportation systems or emergency response, can share and process data in real time. “Organizations must make sure to integrate, process, and analyze data in real-time.”
“Creating data pipelines can simplify the process of making data available in a more accessible and usable format. This can be particularly beneficial for open data initiatives, where the goal is to make data freely available to the public for analysis and innovation,” he adds.
Ultimately, shifts in the open data landscape could have a far-reaching effect on all, from giant corporations to individuals. Therefore, Lherault says, there needs to be metadata associated with the open data. This chain of custody of the data will help us understand how reliable it is. “There needs to be multiple eyes on open data: who created it, who’s changed it, how and when?”
Another risk is a lack of diversity in general. There’s also a risk that people associated with AI presume they can take one element (e.g. a data set or model) and plug it in elsewhere and it will work the same, he adds.
“For example, the New York taxi open data set is well known in data scientist circles. But anyone working with it would have to be careful not to assume that the same journeys would be applicable in other cities. External factors will impact how usable the data is: how each population uses public transport, motorbikes, bicycles, cars, add weather patterns and office working into the equation, and there’s a lot to consider,” says Lherault.
Business Understanding and Technology
While open data can accelerate innovation and its value is amplified when coupled with business understanding and technology to allow users to derive insights faster, experts say additional elements such as contextual comprehension and technological advancements are indispensable.
“Open data has definitely helped to train data scientists. Having access to open data to learn about AI and data science is essential as a prerequisite for innovation,” says Lherault.
“However, it’s not just the data which fuels innovation, it’s the AI models as well. An interesting example is the transportation app Citymapper — it uses open data from multiple sources including government departments. It has brought the open data together to create something new and innovative in an app that is used in many countries worldwide,” he adds.
In addition, it should be remembered that while open data has undoubted value, it doesn’t eclipse the need to mitigate the risk associated with it.
According to Khalife, quality control policies are critical. “Organizations can put in place frameworks to ensure data quality. This includes data validating and cleansing techniques to ensure data meets business requirements. They also implement access control methods to prevent data breaches and misuse of the data.”
The human aspect is crucial when it comes to security, adds Crehan. Employees have to be trained on best practices for data privacy, security, and legal compliance at all times. “Developing a comprehensive risk management plan that outlines strategies for addressing potential risks can also help. By adopting all these strategies, organizations can leverage open data, while maximizing the benefits and minimizing potential risks.”