Reinventing the Organization for GenAI and LLMs
Previous waves of technology have ushered in innovations that strengthened traditional organizational structure. Not so for generative AI and large language models.
News
- How Third-Party Data Can Shape Customer Experiences in Financial Industry
- AI, Data, and IoT Dominate Business Priorities
- These Are the Top Challenges of Middle East CEOs
- UAE releases new AI model to compete with big tech
- How AWS, GCP, and Microsoft Azure Serve the Growing Demand for AI Inference
- AI, Regulation, and Digital Transformation Feature Prominently in the NextTech Agenda
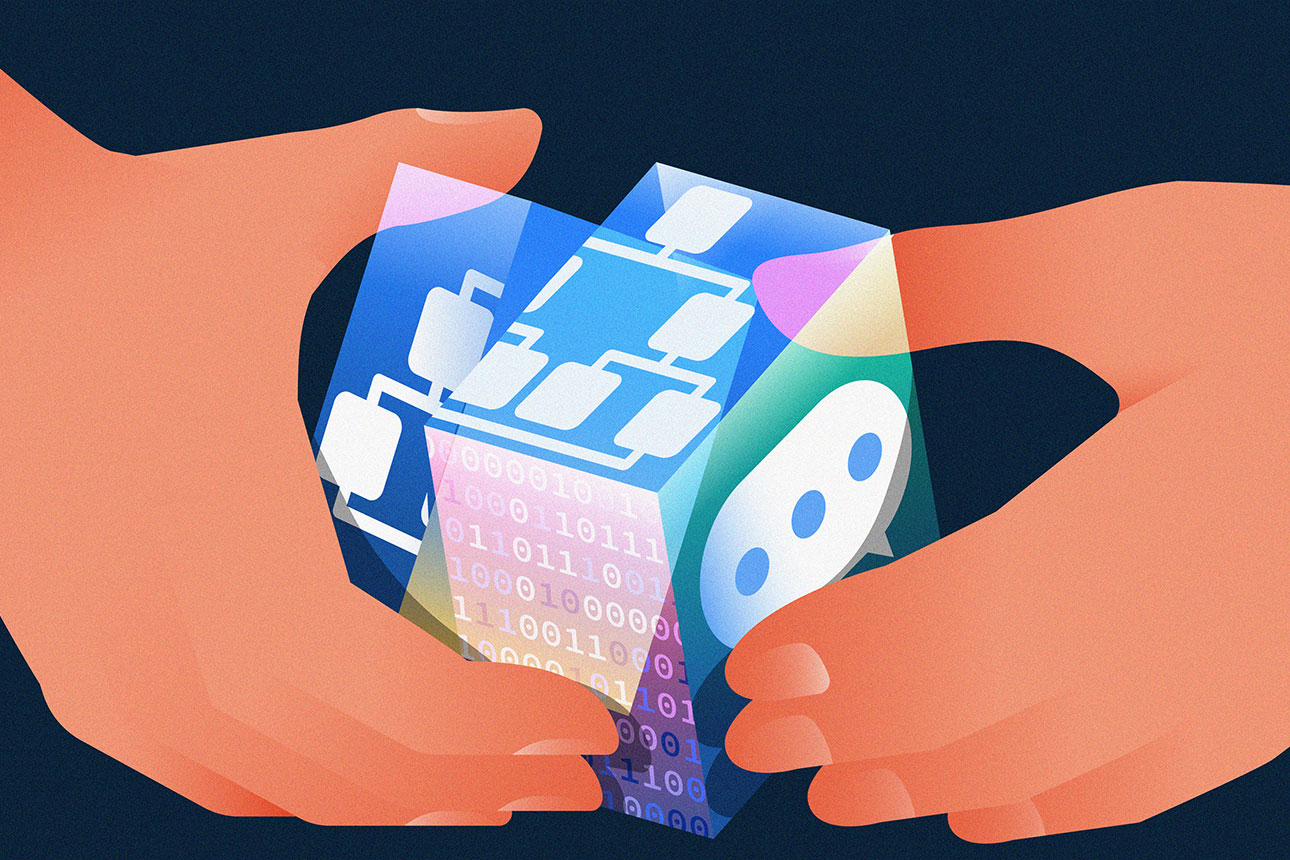
Carolyn Geason-Beissel/MIT SMR | Getty Images
Consider this an early eulogy for the traditional organizational structure, which began in 1855 with the first modern organizational chart and thrived, more or less successfully, until the 2020s, when it succumbed to a new technology, the large language model (LLM).
This is, of course, a bold claim. While traditional approaches to organizing have been frequently threatened by technological advancements (anyone remember the brief craze for holacracy?), organizations have proven remarkably durable. In fact, each new wave of technology ushered in innovations that strengthened traditional organizations. Henry Ford took advantage of advances in mechanical clocks and standardized parts to introduce assembly lines and more precise ways of dividing work. In 2001, agile development took advantage of new ways of working with software and communicating via the internet to revamp how organizations develop products. Technology breakthroughs and organizations have long been partners.
But generative AI, and the LLMs that power it, are different. Every previous method of organizing was intensely human, built on human capabilities and limitations. That is why traditional organizational models have persisted for so long. Human attention remains finite, so we needed to delegate our tasks to others. The number of people who can work in a team is limited, so we needed to break organizations into smaller parts. Decision-making is complicated, so we embraced layers of management and authority. The technology changes, but workers and managers are just people, and the only way to add more intelligence to a project was to add people or make them work more efficiently through tools that helped them communicate or speed up their work.
But this is no longer true. Anyone can add intelligence, of a sort, to a project by including an AI. And evidence shows that people are already doing so — they just aren’t telling their bosses about it: A fall 2023 survey found that over half of people using AI at work are doing so without approval, and 64% have passed off AI work as their own.
This shadow AI use is possible partly because LLMs are uniquely suited to handling organizational roles — LLMs work at a human scale. Tools based on LLMs can read documents and write emails and adapt to context and assist with projects without requiring users to have specialized training or complex, custom-built software. While large-scale corporate installations of LLMs have some advantages, like data privacy and data integration, people with access to ChatGPT-4 can just start having AI do work for them. And they are clearly doing just that.
AI and LLMs’ Impact on Organizational Process
What does it mean for organizations when we acknowledge that this behavior is happening? We face the same challenge the original railroad operators did 150 years ago: how to rebuild an organization around a fundamental shift in the way work is done, organized, and communicated.
I have already experienced some of this at Wharton Interactive, a small software startup inside of the Wharton School of the University of Pennsylvania devoted to transforming education through AI-powered simulations. Given our mission, we embraced the power of LLMs early on. Our customer support team uses AI to generate on-the-fly documentation, both in our internal wiki and for customers. Our CTO taught the AI to generate scripts in the custom programming language we use (a modified version of Ink, a language for interactive games). We use our AI tools to add placeholder graphics, to code, to ideate, to translate emails for international support, to help update HTML in our websites, to write marketing material, to help break down complex documentation into simple steps, and much more.
We have effectively added multiple people to our small team, and the total compensation of these virtual team members is less than $100 a month in ChatGPT Plus subscriptions and application programming interface costs.
But, in many ways, this is just the start. We are considering how to completely change organizational processes. We have learned that we can have AI act as a software tester for initial feedback on our designs (research shows that you can get reasonably good simulated feedback from AI personas). AI can record and combine our ideas with relatively little human oversight so that we can skip some meetings, and it can provide guidance to keep the meetings we do have on track. And those meetings can focus on building, not just planning. Instead of a long session of compiling information, we can have the AI create web pages and images based on our ideas. We aren’t just meeting; we are instantly able to ask for changes and see a prototype of the web page results, even without coding experience.
The changes are profound. Theoretical discussions become practical. Drudge work is removed. And, even more importantly, hours of meetings are eliminated, and the remaining meetings are more impactful and useful. A process that used to take us a week can be reduced to a day or two. And these are just off-the-shelf projects built with GPT-4, not the most imaginative version of this sort of future. We already can envision a world where autonomous AI agents start with a concept and go all the way to code and deployment with minimal human intervention. This is, in fact, a stated goal of OpenAI’s next phase of product development. It is likely that, if GenAI’s hallucination rates decrease in future versions, entire tasks can be outsourced largely to these agents, with humans acting as supervisors.
Controlling Versus Coaching: Your Decision
Of course, the future of AI-filled organizations could head in many directions. By managing work, or at least by helping managers manage work, the enhanced capabilities of LLMs invite radical change in ways that can be either positive or negative. A single AI can talk to hundreds of workers, offering advice and monitoring performance. AI tools could mentor — or they could manipulate. These tools could guide decisions in ways that are overt, or subtle.
Companies have been experimenting with forms of computerized control over workers since long before this generation of AI. Time clocks, cameras, and other forms of monitoring have been common for over a century. But these approaches kicked into high gear with the rise of pre-LLM AI tools, particularly the use of algorithms to control work and workers.
Think of the gig worker hoping that Uber will give them a good stream of customers, despite receiving a low rating from an angry passenger. Picture the UPS driver whose every minute of driving is scrutinized by an algorithm to see if they are efficient enough to keep their job. Katherine Kellogg, the David J. McGrath Jr. (1959) Professor of Management and Innovation at MIT Sloan School of Management, along with Melissa Valentine and Angèle Christin, both Stanford University professors, outlined how these new types of control were different than previous forms of management. Where previously managers had limited information about what workers were doing, algorithms supply a comprehensive and almost instantaneous picture, using massive amounts of data from many sources to track workers. These algorithms also work interactively, channeling workers in real time to whatever task the company wants. And the algorithms are opaque — their biases and even the way they make decisions are hidden from workers.
We could imagine how LLMs might supercharge this process, creating an even more comprehensive (and worrying) panopticon: In this type of system, every aspect of work is monitored and controlled by AI. AI tracks the activities, behaviors, outputs, and outcomes of workers and managers. AI sets goals and targets for them, assigns tasks and roles to them, evaluates their performance, and rewards them accordingly. But, unlike the cold, impersonal algorithm of Lyft or Uber, LLMs might also provide feedback and coaching to help workers improve their skills and productivity in ways that many humans would find warm and persuasive. AI’s ability to act as a friendly adviser could sand down the edges of algorithmic control, covering the Skinner box in bright wrapping paper. But it would still be the algorithm in charge. If history is a precedent, this is a likely path for many companies, which often see technology as a way to exert more control over workers.
But other, more utopian possibilities also exist. We don’t need to subject vast numbers of humans to machine overlords. Rather, LLMs could help us flourish. There are hints buried in the early studies of AI about a way forward. Workers, while worried about AI, tend to like using it when it removes the most tedious and annoying parts of their job, leaving them with the most interesting tasks. AI-centered organizations could become more meaningful and more high-value to engaged employees, supporting talent retention.
This is not inevitable, however, so managers and leaders must decide whether and how to commit themselves to reorganizing work around AI in ways that help rather than hurt their human workers. You need to ask: What is your vision about how AI makes work better rather than worse?
Three Principles for Reorganizing Work Around AI
Managers need to start taking an active role in answering that question. Like everything else associated with AI, there is no central authority that can tell you the best ways to use AI — organizations will need to figure it out for themselves. Use these leadership principles to guide your thinking:
1. Identify and enlist your current AI users. As noted earlier, many people are using AI but hiding it from their own managers. The best way for an organization to move forward with AI is to get the help of these workers. And that is going to require a major change in how organizations operate. Leaders need to recognize that the employees who are figuring out how to best use AI may be at any level of the organization, with varying histories and performance records.
In other words, your company’s AI skills might be anywhere. Right now, there is some evidence that the workers with the lowest skill levels are benefiting most from AI and so might have the most experience in using it, but the picture is still not clear. As a result, companies will need to include as much of their organization as possible when building their AI plans. They will need to provide broad training to these workers and offer tools to help them share lessons broadly with the team, such as by creating crowdsourced ChatGPT prompt libraries.
However, to make this approach work, leaders need to figure out a way to decrease the fear associated with revealing AI use. Leaders can minimize anxiety that employees will be laid off as a result of AI use, or promise that workers will be able to use the time gained via AI to work on more interesting projects, or even use the time to improve work-life balance.
If your employees don’t believe that you care about them, they will keep their AI use hidden. And you will need to incentivize them to come forward. That means not only permitting AI use but also offering substantial rewards to people who find substantial opportunities for AI to improve work processes. Think substantial cash prizes. Promotions. Corner offices. The ability to work from home forever. With the potential productivity gains possible due to LLMs, rewards are a small price to pay for truly breakthrough innovation. And large incentives also show that the organization is serious about this issue.
2. Let teams develop their own methods. All of the usual ways organizations respond to new technologies don’t work well for AI. These approaches are all far too centralized and slow. The IT department cannot easily build an in-house AI model, and certainly not one that competes with one of the major LLMs. Many CIOs are taking control of managing AI for security reasons while dealing with the fact that some workers may hide their AI use. Consultants and systems integrators have no special knowledge about how to make AI work for a particular company, or even the best ways to use AI overall. The innovation groups and strategy councils inside organizations can dictate policy, but they can’t figure out how to use AI to actually get work done — only work teams can do that.
And, given that AIs perform more like people than software (even though they are software), they are often best managed as additional team members rather than external IT solutions imposed by management. Teams will need to figure out their own ways to use AI through ethical experimentation, and then they will need a way of sharing those methods with each other and with organizational leadership. Incentives and culture will need to be aligned to make this happen, and guidelines will need to be much clearer for employees to feel free to experiment, within guidelines set for security and governance reasons.
3. Build for the not-so-distant future. Everything I have discussed is already possible today using ChatGPT-4. But more advanced models are coming — and coming fast. Organizational change takes time, so organizations adapting processes to AI should do so while considering future versions of AI rather than just building for today’s tech. If the sort of efficiency gains we are seeing from early AI experiments continue, organizations that wait to experiment will fall behind very quickly.
If you truly can trim a weeks-long process into days, that is a profound change to how work gets done, and you’ll want your organization to get there first or at least be ready to adapt to the change. You should also be building and planning with the mindset that the tools will evolve rapidly. That mindset doesn’t favor top-down solutions that take months or years to implement.
There are only two ways to react to exponential change: too early or too late. Today’s AI tools are flawed and limited in many ways. While that restricts what AI can do, the capabilities of AI are increasing exponentially, both in terms of the models themselves and the tools these models can use. It might seem too early to consider changing your organization to accommodate AI, but there is a strong possibility that it will quickly become too late.