Navigating Responsible Implementation of Data Ethics in the Age of AI
Leaders must be armed with the right tools and knowledge to navigate the ethical implications concerning data to avoid biases .
Topics
News
- Manufacturing Industry Will Reach 108 Million Private Cellular IoT Connections in 2030
- Hyperscale Data Centers Growing at an Impressive Pace, Driven by Generative AI
- Data & Analytics Summit to Unlock the True Value of Data
- Innovate, or Lose Your Seat at the Top Table
- Unlocking Insights into Data Warehousing and Data Mining Trends
- Responsible AI Governance: How to Balance Innovation with Ethical Use
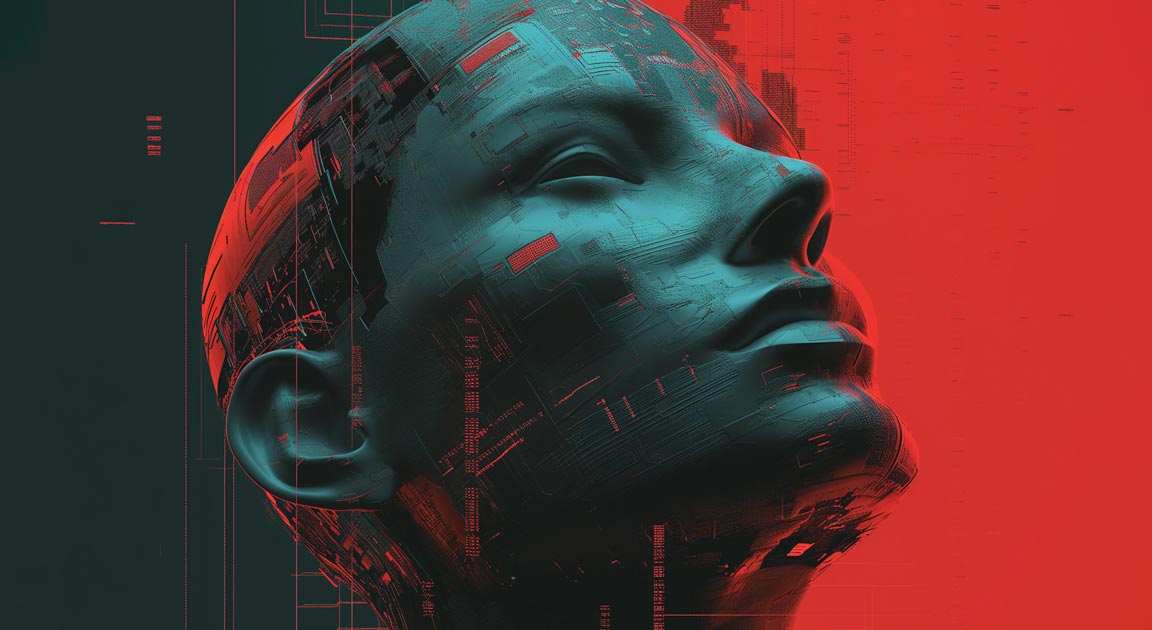
MITSMR Middle East Design
Artificial Intelligence (AI) systems undoubtedly have the potential to unlock efficiencies within business operations. But the tool can be a double-edged sword, owing to the ethical implications that may arise related to the management of data – something that leadership within organizations must be vigilant in navigating.
Trustworthiness and responsibility are important for AI development and deployment. “Setting a foundation of ethical AI practices will be key to implementing successful business use cases, especially as Generative AI continues to unlock new developments in enterprise applications,” says Triveni Gandhi, Responsible AI Lead at Dataiku.
AI has real implications for people. It can reflect or even worsen existing biases against people of certain gender identities, races, ethnicities, and so on.
“AI can be trained on historical data that contains biases, so when it comes to deployments such as in AI-based credit scores, there could be biases towards certain groups that perpetuate long-term financial inequality,” says Christoph Wollersheim, a member of Egon Zehnder’s Services Practice and Artificial Intelligence practice.
Ethical data management practices in the context of government institutions are critical. “In the defense sector,” Wollersheim says, “When AI algorithms are being used in detecting targets or being used in driverless vehicles on the battlefield, these are life and death decisions. The question is, how do you ensure you are implementing a responsive yet trustworthy AI system?”
Navigating The Challenges
Some approaches to putting AI ethics into practice, Gandhi says, include setting up processes for accountability and transparency over all AI projects, developing models with bias and fairness issues in mind, and monitoring pipelines in production.
However, understandably, this is easier said than done. Challenges vary based on how AI is deployed. “The first is an ‘out of the box’ solution using Open AI,” says Felicien Mathieu, Chief Technologist EMEA, World Wide Technology. “The challenge here is a lack of business knowledge; the AI doesn’t fully understand the business data, and uploading your IP is a risk.” In this scenario, Mathieu says businesses often don’t fully understand how to deploy AI, let alone the ethical considerations.
The second typical deployment is fine-tuning existing AI systems with company data. “This is where we see most customers right now,” he says. “The challenge here is integration. Businesses must be aligned internally, including stakeholder engagement, from data teams to security. Deploying this platform from scratch is a complex process that requires reference architectures and blueprints for GenAI.”
The third type, Mathieu says, is businesses building their AI from scratch. These are generally larger corporate entities with significantly bigger budgets, often over $100 million. “As they implement AI, the challenge is deploying a safe system from day one, which requires careful curation of datasets to avoid sharing personal information and potential bias.”
Across different types of deployments, two main concerns arise. The first relates to the complicated task of ensuring ethical data collection and consent. “Foundational models are trained on vast datasets, making it challenging to ascertain whether the data used for training and analysis was sourced and managed ethically,” says Jad Haddad, Head of Digital, MEA, Oliver Wyman. Secondly, there is a lack of transparency when AI models generate results in a “black box” manner, offering little explanation for the outcomes. “This opacity, prevalent in models with trillions of parameters, can lead to flawed decision-making,” Haddad says, pointing to the risk of erroneous actions stemming from results that are insufficiently explained.
The ethical landscape of AI and data is fraught with challenges. Hence, protecting the organization’s privacy is essential and requires tight security to defend against unauthorized access and guard the safety of both company and client data. “Data quality, too, is critical. Errors from manual inputs or system glitches can lead to incorrect or incomplete data. Such lapses open doors to bias, where skewed or selective information influences outcomes and decisions,” says Dr. Mishal Taifi, a corporate AI champion.
What Does it Mean to be Adaptive and Responsive?
Given the rapidly changing AI environment, companies must be adaptable and responsive, particularly with the emergence of GenAI, as enterprises move into industrial-scale development and deployment.
“To scale AI responsibly, organizations must first set their goals and expectations for AI, and boundaries on what kind of impact they want AI to have on their customers and users, as a foundation,” Gandhi says. “From there, these values can be translated into actionable criteria and acceptable risk thresholds.”
A tailored approach is critical. As effective use of AI enables organizations to be relevant and ensure a competitive advantage, cultural changes among leadership and the board’s involvement are paramount. “We advise companies to have an AI review board, consisting of AI practitioners and representatives from the business side to facilitate monthly check-ins and mitigate risk within the organization,” says Wollersheim.
Robust governance mechanisms ensure organizations uphold pre-established values and align with societal needs and business goals are important. Building ethical AI practices is a comprehensive effort that requires open dialogue and collaboration to minimize bias and ensure equitable outcomes.
Strategies in Practice
There is a strong move in this direction, with Mathieu reporting a sharp rise in AI consulting services. “100% of our customer base now requires a strategy for responsibly and ethically deploying AI.”
In practice, this means an AI manifesto that includes the specific rules of engagement and guardrails the business needs to implement to deploy the right system, generate safe data, and robust training at key organizational touchpoints.
Finding the proper equilibrium between gleaning insights from data and adhering to ethical standards means employing strong data security tactics, such as tokenization and anonymization.
Ongoing education, working alongside regulatory authorities, and using AI to track compliance help embed a culture of ethical AI usage.
“At the end of the day,” says Wollersheim, “it’s about figuring out what you have the right to do and what is right to do – that’s what data ethics is.”
Keen to know how emerging technologies will impact your industry? MIT SMR Middle East will be hosting the second edition of NextTech Summit.