Is Legacy AI Dead? Experts Discuss the Future Amidst the Rise of Gen AI
Experts highlight that successful AI adoption depends on knowing when and where to deploy these technologies.
Topics
MIT SMR CONNECTIONS
News
- More than 80% of Saudi CEOs adopted an AI-first approach in 2024, study finds
- UiPath Test Cloud Brings AI-Driven Automation to Software Testing
- Oracle Launches AI Agent Studio for Customizable Enterprise Automation
- VAST Data and NVIDIA Launch Secure, Scalable AI Stack for Enterprises
- How Machine Identity Risks Are Escalating in AI-Powered Enterprises
- How Agentic AI Is Reshaping Healthcare
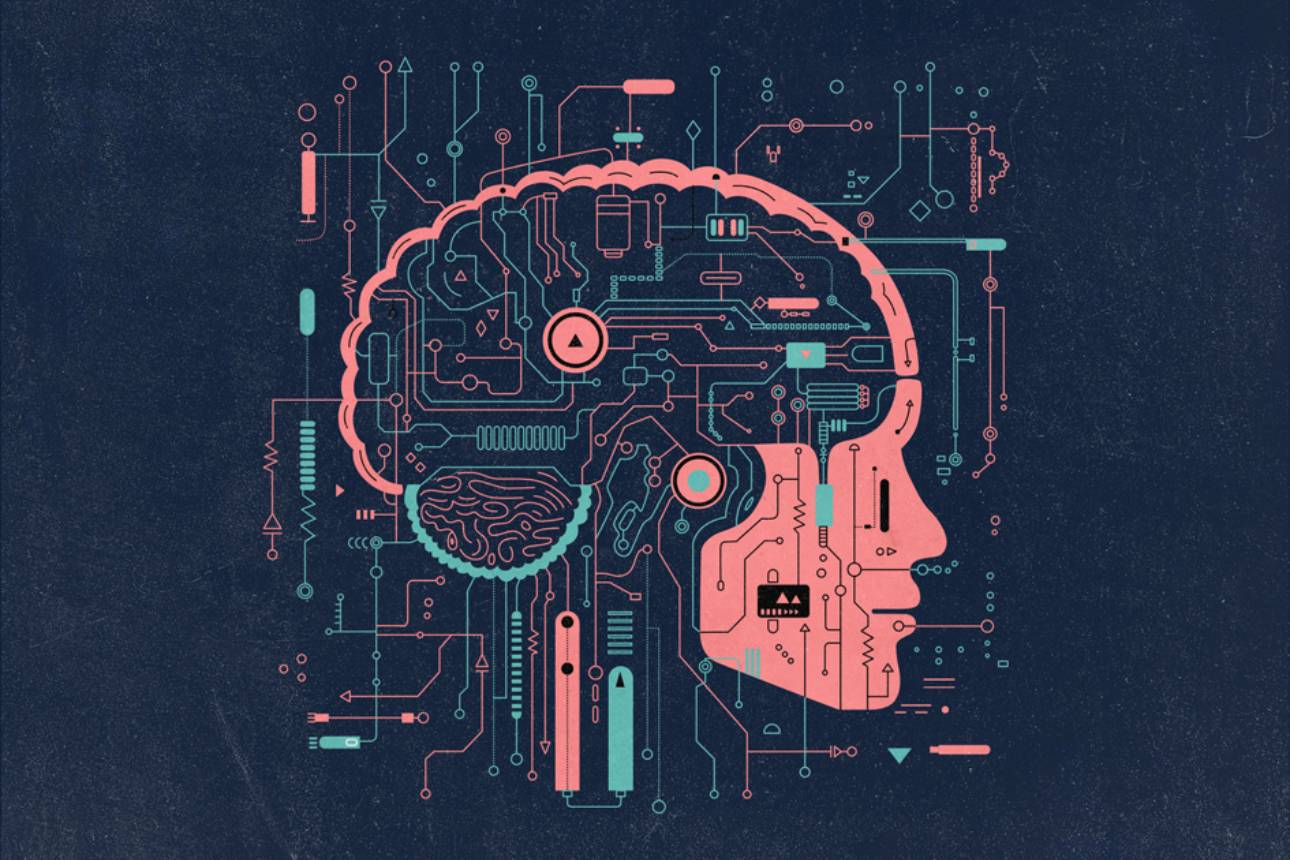
[Image source: Krishna Prasad/MITSMR Middle East]
It was a usual May evening in Dubai, a city known for its relentless pursuit of technological innovation and digital transformation. As so often these days, the topic of discussion was artificial intelligence—a conversation that continues to dominate news, summits, and boardrooms alike and is mostly likely to do so for years to come.
But this was no ordinary discussion. In the room were chief technology officers, data scientists, analysts, and data architects—the very minds executing AI strategies within their organizations.
The central question on the table: Is legacy AI dead?
These experts were asked to vote on what appeared to be a straightforward question, one that seemed to demand nothing more than a simple “yes” or “no.”
But is the answer truly that simple?
For one, legacy systems are called “legacy” for a reason—they’re the foundational structures on which modern technologies are built, proven reliable, and trusted over time. So, why is there a sudden rush to embrace Generative AI (GenAI), a technology that’s only recently begun to dominate the conversation? The answer isn’t black and white for organizations heavily invested in existing infrastructure. Finding the right path forward requires careful consideration, especially when so much is at stake.
Current Limitations and Risks with Gen AI
According to the Lucidworks report, 58% of manufacturing leaders plan to increase AI spending in 2024, down from 93% in 2023. One of the most significant barriers to widespread adoption is trust in the accuracy of AI-driven recommendations. The report found that 44% of manufacturers have concerns about response accuracy.
Experts believe tempering the excitement with a grounded understanding of the technology’s current limitations and potential is essential.
“Gen AI does not have limitations; the pressure of the market created too many expectations that the technology cannot meet in the short term. It will grow and mature, but as with anything, it will need time. The foundation models currently in place need enrichment and recency, which is not quick,” says Dr. Mohamed Elfarra, Head of Data Insights and Advanced Analytics for Automotive at Al-Futtaim.
From a commercial perspective, Dr. Elfarra says if he had $10 to invest, he would allocate $8 to Legacy AI (LAI) and only $2 to Gen AI at this stage. The reason he adds is straightforward: Gen AI is currently a race to the bottom. It relies heavily on a few foundational models, owned by a limited number of organizations. These companies have the power to disrupt any third-party investments by offering their models for free or at low cost, making it risky to allocate significant resources to Gen AI prematurely.
Emphasizing that Gen AI is transforming multiple industries, Dmitriy Dovgan, former Head of Data Science at Al-Futtaim, says, “The current limitations justify continued investment in LAI.”
One of the key challenges with Gen AI is its tendency to produce unreliable or incorrect outputs—hallucinations. “Gen AI often cannot learn independently, lacks in-depth human creativity, struggles with complex contexts, and can be difficult to adapt to specific business needs in sectors like finance and healthcare,” Dovgan explains. Moreover, the models are resource-intensive, requiring vast amounts of data and computational power, which can create scalability concerns for organizations with limited resources.
In contrast, traditional AI still outperforms Gen AI in anomaly detection, optimization, regression, classification, and forecasting tasks. “Specific, fine-tuned algorithms are more efficient for these tasks,” Dovgan points out. It offers stability, predictability, and better interpretability, crucial for operations like warehouse operations optimization, sales forecasting, supply chain management, etc.
Although both can involve black-box models, LAI offers better interpretability in many cases, making it more suitable for industries requiring more precise decision-making paths. Interpretability remains a significant constraint for Gen AI; its black-box nature limits trust and adoption in highly regulated industries.
Dovgan also cautions against a complete shift from LAI to GenAI. “Shifting entirely from LAI to Gen AI is not feasible today,” he adds. Organizations risk losing valuable domain expertise embedded in LAI and incurring significant costs for the transition. Additionally, the high computational resources and infrastructure costs for Gen AI can disqualify small and medium-sized organizations from a competitive landscape.
Dr. Elfarra agrees with this approach. He emphasizes the risks inherent in adopting new and unstable technology. “Like any new technology, risks will be related to over-reliance, and expectations will be missed as the new technology is still evolving and unproven.”
Another concern is the disruption of existing methodologies and operations. Prior to adopting any new technology, a lot of integration homework needs to be done. At the current pace, Dr. Elfarra says, too many shortcuts will halt most activities.
As businesses navigate the complexities of AI adoption, understanding when and where to deploy these technologies will be vital to achieving sustainable success.
A Synergistic Approach
While LAI has been a cornerstone of many industries for years, GenAI is rapidly emerging as a powerful tool with the potential to revolutionize a wide range of applications. Rather than viewing it as a replacement for traditional AI, experts suggest a more integrated approach.
“We don’t believe there is an ‘either/or’ scenario with Gen AI and LAI,” says Dael Williamson, CTO at Databricks EMEA. “Instead, we foresee a future where a compound AI system emerges—one that combines the strengths of conventional and modern AI, and other advanced computer science techniques. This fusion will enable organizations to tackle more complex problems and make more adaptive and informed decisions.”
Shashank Dubey, Co-founder of Tredence Inc., also supports this integrated approach. “We continue to invest in both traditional forms of AI and GenAI,” he notes. “Organizations should not overlook the value that can be realized from regressions, prediction AI, etc. Our customers need certainty about the nature of the model, the data it has ingested, and what to expect. This trust factor is invaluable.”
Echoing these sentiments, Dr. Elfarra advocates for an incremental approach to technology adoption. “As with any new technology adoption curve, you need to start by enhancing, not replacing. The future will be a hybrid model-building exercise where modelers will be given a working AI-generated method, code, or model and tasked with improving its performance.”
Dovgan recommends a comprehensive three-phase hybrid approach for organizations looking to effectively adopt and integrate LAI and Gen AI technologies.
Phase-1: Exploration
- Identify areas where Gen AI can complement or enhance existing LAI solutions.
- Design a hybrid AI architecture that integrates legacy and Gen AI models seamlessly.
- Implement Gen AI in smaller, non-critical use cases and gradually expand its role by showcasing its effectiveness.
Phase-2: Scaling and Optimization
- Introduce a robust AI governance framework to manage risks and biases, ensure ethical AI practices, and maintain transparency.
- Invest in data harmonization to ensure compatibility between technologies.
- Build a skilled team capable of managing both technologies.
- Evaluate the performance and apply the required adjustments to optimize LAI and Gen AI.
Phase-3: Continues Improvement
- Monitor legacy and Gen AI performance and allocate resources accordingly.
- Implement Gen AI in more critical use cases, ensuring it complements and does not replace LAI where stability is needed.
- Continuously update the AI governance framework to consider new risks, legal standards, and emerging ethical considerations.
Role-Specific Gen AI Strategies
Christopher Wallace, Regional Managing Director, Middle East at Tredence Inc., recommends an approach that is tailored to the role within the ecosystem, whether you’re a business leader like a Chief Data & Analytics Officer (CDAO) or Chief Technology Officer (CTO), a Data & AI Vendor, or a ‘doer’ in the trenches, such as an engineer or data analyst.
“Should you go full tilt on Gen AI? It really depends on the persona,” states Wallace.
For business leaders, the stakes are higher than ever. Traditionally, these leaders have been responsible for steering their companies through the complexities of digital transformation. However, with the rapid emergence of GenAI, they are now facing increased pressure from boards and senior management to justify investments in this cutting-edge technology. It’s no longer a matter of maintaining the status quo; they must now critically assess the potential of GenAI to drive business outcomes. Given this responsibility, it’s advisable for them to “deploy a small proof of value and assess the impact.” This allows them to experiment with GenAI’s capabilities on a smaller scale, measure its impact, and determine whether a larger-scale implementation is warranted. By taking this cautious yet strategic approach, business leaders can manage risk while positioning their organizations to leverage the transformative power of GenAI.
On the other hand, data and AI vendors, whose business models have traditionally relied on established AI technologies, face a different challenge. While their revenue streams are largely tied to conventional AI, they cannot ignore the growing demand for GenAI solutions. In this competitive landscape, Gen AI is not just a trend; it represents a fundamental shift in how AI can be applied to solve complex business problems. Vendors should strategically “bet big” in select areas where they believe Gen AI can offer the most value. This might involve developing specialized Gen AI products, investing in research and development, or forming strategic partnerships that enable them to provide innovative solutions. However, these investments should be targeted rather than broad, ensuring they align with the vendor’s core competencies and market demands.
For the “doers”—the engineers and analysts who are directly involved in implementing and optimizing AI technologies—the message is clear: “Go all in or become obsolete.” As AI continues to evolve, the skills and knowledge that were once sufficient may no longer be enough. The rapid advancement is reshaping the landscape, and professionals in this space must adapt quickly. This means acquiring new skills, staying up-to-date with the latest Gen AI tools and techniques, and being willing to experiment and innovate. For these practitioners, embracing Gen AI is not just an option; staying relevant in an increasingly competitive job market is necessary.
LAI Applications and Enhancements with Gen AI
At Al-Futtaim, conventional AI applications have proven invaluable across various operational areas.
One notable example, Dovgan highlights, is a sales forecasting model developed for one of Al-Futtaim’s retail brands. This model uses historical sales and inventory data to forecast seasonal orders up to nine months in advance. This capability simplifies order planning and preparation, reduces lost sales opportunities, and lowers inventory holding costs.
According to Dovgan, “Gen AI can enhance this forecasting model by improving data quality through synthetic data generation and creating new features. Additionally, with Generative AI, we can generate data scenarios for unexpected events, such as supply chain disruptions or sudden market shifts, allowing the business to be more agile and responsive in its decision-making.”
Dr. Elfarra describes several internal applications of LAI within Al-Futtaim:
- Customer Centricity: Includes customer segmentation, lead scoring, next-best car model recommendations, and retention/churn prevention.
- Supply & Range Management: Focuses on sourcing and optimal used car mix solutions.
- Predictive Modeling: Encompasses demand forecasting and service date predictions.
However, advancements are also evident in areas traditionally handled by LAI. For instance, verbatim/sentiment analysis is fully automated using Gen AI. The ongoing developments in Al-Futtaim’s mini digital lab involve merging the two AI paradigms. The process begins with Gen AI for ideation and conceptualization, followed by optimization and enhancement by the data science team. This hybrid approach saves time and money and reflects a new trend in leveraging both models to create effective solutions.
Dovgan says the Blue Rewards App, an Al-Futtaim loyalty application, is an excellent example of the effective synergy between traditional and modern AIs.
The data scientist explains the process involved: This application features an AI search functionality that significantly enhances offer discovery. This solution seamlessly integrates a robust recommendation engine that analyzes customer interaction data to predict preferences based on past behavior and purchase history. Gen AI enhances the search experience by enabling users to employ natural language queries. The model intelligently parses these requests, leveraging its understanding of context and semantics to pinpoint relevant offers from a comprehensive knowledge base. This powerful combination elevates user satisfaction and significantly improves offer redemption rates and overall customer engagement.
Solution-Oriented Approach
Tredence, a company specializing in industry-specific data analytics solutions, addresses what they term the “last mile problem” in AI. This challenge refers to the gap between insight creation and value realization.
Unlike solutions aimed at CEOs or CIOs, Tredence focuses on empowering front-line decision-makers, says Dubey. For instance, the data science solutions are designed to help shop floor employees optimize production, assist store managers with inventory management, enable supply chain executives to forecast demand with greater accuracy, and provide health workers with actionable patient recommendations. This approach is embodied in ATOM.AI, Tredence’s integrated accelerator ecosystem, which supports enterprises from the design phase to experience and value generation.
As the boardroom discussion drew close, a delegate light-heartedly referenced Spike Jonze’s Her, suggesting that humanity might harmoniously coexist with technology one day. The room chuckled, reflecting on a future where such synergy might become a reality. However, the transition to Gen AI is not an immediate necessity for everyone today. The consensus among the delegates was clear: Legacy AI still holds substantial value.
As Dubey aptly puts it, “Until Gen AI answers the ‘why?’, most businesses will find greater benefit in continuing to leverage traditional AI approaches.”
The experts were part of the MIT SMR CONNECTIONS thought leaders briefing “Is Legacy AI Dead?” powered by Tredence. At MIT SMR Connections, we explore the latest trends in leadership, managing technology, and digital transformation.